Exploring Meena: Google's Conversational AI Breakthrough
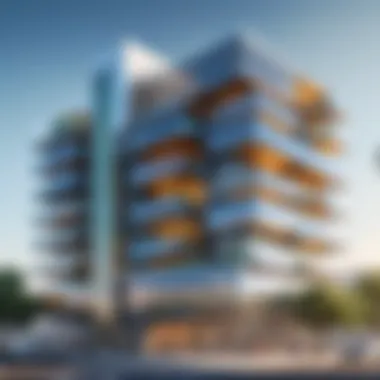
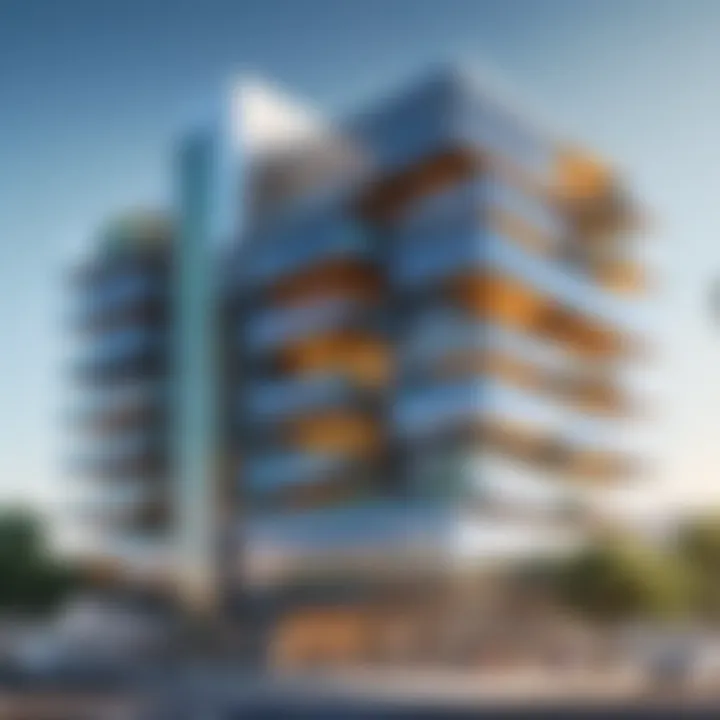
Intro
In the rapidly evolving landscape of artificial intelligence, Google AI has made significant strides in developing conversational agents capable of engaging in nuanced dialogues. This article delves into Meena, a groundbreaking model that represents a leap forward in open-domain conversation. Throughout this exploration, we will dissect Meena's architectural design, data training techniques, and its performance metrics, while also contemplating its implications across various sectors. Understanding Meenaโs potential applications in fields like customer service and virtual assistance can reveal much about the future direction of conversational AI.
Overview of the Technology
Meena is designed as an end-to-end neural conversational model, which makes it quite different from traditional techniques. Its architecture employs a sophisticated approach to natural language understanding and generation, allowing it to create more relevant and engaging responses.
Key Specifications
- Architecture: Meena integrates a 2.6 billion parameter model, which considerably enhances its ability to understand context and generate appropriate responses. This scale allows for more expressive dialogues.
- Training Data: The model has been trained on a diverse set of conversations scraped from various sources, encompassing a wide variety of topics. This extensive dataset ensures Meena can handle intricate discussions across numerous domains.
- Performance Metrics: It was evaluated based on several benchmarks, including the Sensibleness and Specificity Average (SSA), achieving a score significantly higher than previous models. This metric measures how sensible and specific Meena's responses are during interactions.
Unique Selling Points
One of Meena's standout features is its ability to maintain conversational context over a longer span of dialogue. Unlike other models that may lose track after a few exchanges, Meena can remember past interactions within the same conversation, contributing to a more human-like experience.
Additionally, the model's proficiency in generating diverse responses minimizes repetitive dialogue. This quality is crucial in retaining user engagement and creating a satisfying interaction experience.
"Meena's conversational abilities open doors to applications that extend beyond mere task completion, delving into user engagement in meaningful conversations."
Challenges and Considerations
While Meena's capabilities are impressive, it is important to recognize the challenges that come with such advanced systems. Topics like safety, bias in data, and ethical use remain critical concerns. Ensuring that conversational agents like Meena operate within ethical guidelines and do not reinforce negative behavior or bias calls for ongoing scrutiny and adjustment.
As we proceed through this discussion, we will further analyze how Meena stands against existing conversational AI technologies and what this means for its future in various applications.
Prelims to Meena
Meena represents a significant shift in the domain of conversational agents and artificial intelligence. As an advanced model developed by Google AI, it highlights not only the technical progress made in natural language processing but also the intricate challenges that arise in creating machines capable of understanding and responding to human language. The introduction of Meena strikes at the core of what conversational AI could achieve, emphasizing the need for increasingly sophisticated systems capable of engaging in open-domain conversations.
Definition of Meena
Meena is an end-to-end neural conversational model. Its architecture is designed to facilitate dynamic and engaging dialogues between users and machines. Unlike previous models that often relied on limited and structured responses, Meena aims to understand the context and intent behind conversations. This holistic approach allows it to generate responses that are more relevant and nuanced. By integrating various training techniques, Meena stands out in its capacity to manage open-domain conversations, making it applicable in a range of scenarios, from personal assistants to customer service agents.
Significance in AI Development
The development of Meena marks a vital step in the ongoing evolution of artificial intelligence. Its significance is multifaceted:
- Improving Conversation Quality: Meena's ability to produce coherent and contextually relevant responses pushes the boundaries of what conversational AI can do. Improved conversational quality directly influences user satisfaction.
- Broad Applications: The flexibility of Meena offers numerous applications. Sectors like healthcare, finance, and education can benefit from AI-driven conversational interfaces that can handle diverse queries and provide assistance.
- Ethical Considerations: As Meena evolves, it brings about discussions on ethical implications in AI design. Issues like data privacy, user manipulation, and bias must be addressed to advance responsible AI development.
"In a world increasingly driven by technology, innovations such as Meena illuminate the path toward more natural human-computer interactions."
In summary, Meena exemplifies the stride toward creating conversational agents that not only respond but also engage intelligently. Its definition and significance within AI development highlight the potential it holds for reshaping interactions in various fields.
The Evolution of Conversational AI
The journey of conversational AI is a fascinating narrative of technological progress. This evolution has not only shaped the way machines interact with humans but has also influenced various industries, transforming customer service, entertainment, and personal assistance. Understanding this evolution provides context for appreciating Google AI's Meena and its capabilities.
Historical Context
The origins of conversational AI trace back to the early attempts at creating machines that could mimic human conversation. In the 1960s, the development of simple rule-based systems like ELIZA marked the beginning of this journey. ELIZA interacted with users by recognizing certain keywords and responding accordingly, albeit in a very superficial manner. It was significant because it opened the door to the concept of machine dialogue, but the limitations were clear.
Fast forward to the 1990s, advances in natural language processing (NLP) led to the creation of more sophisticated models. Systems began utilizing larger databases and incorporating basic machine learning techniques. However, these models still struggled with context and coherence in conversation. The 2010s brought major milestones with the introduction of deep learning. Breakthroughs in neural networks enabled systems to learn from vast amounts of text data. Notable examples include IBM's Watson, which gained fame for winning the quiz show Jeopardy!, and Google's own advancements in speech recognition and AI language models.

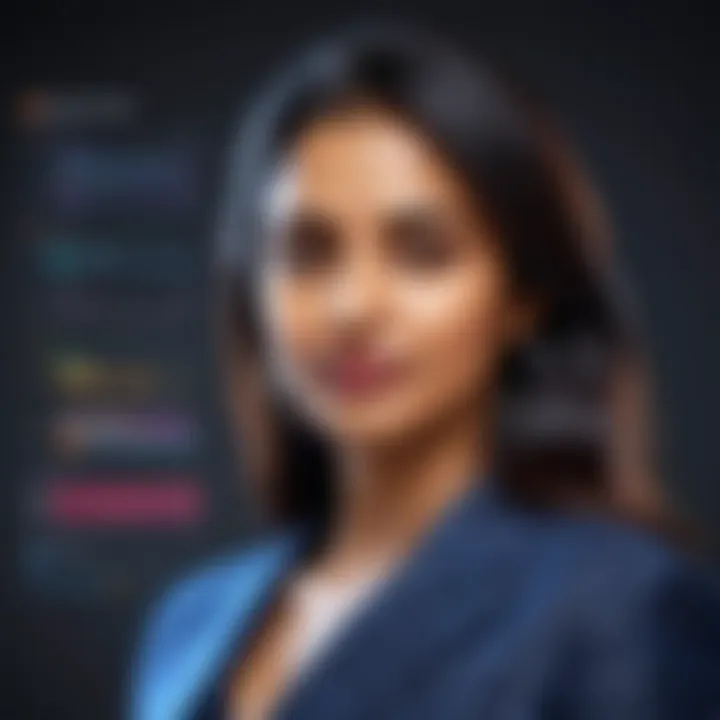
Current Trends
Today, conversational AI is experiencing rapid growth and transformation. Some key trends stand out:
- End-to-End Systems: Modern systems, like Meena, are increasingly designed as end-to-end solutions, processing input and generating output in one continuous flow. This contrasts with previous models that relied heavily on distinct components for understanding and response.
- Contextual Understanding: The focus is shifting towards enhancing context-awareness in conversations. This means developers are prioritizing models that can remember previous interactions within a session and adapt responses accordingly.
- Multimodal Interactions: Increasingly, conversational agents are expected to handle multiple forms of communication, such as text, voice, and even visual inputs, creating richer interactive experiences.
- Personalization: More emphasis is being placed on tailoring conversations to individual users. Leveraging machine learning and historical data allows models to meet user preferences and improve the relevance of interactions.
In summary, the evolution of conversational AI illustrates a trajectory from simple rule-based systems to advanced neural models capable of engaging in meaningful discourse. The historical context lays a foundation for understanding contemporary trends, setting the stage for innovations like Meena that promise improvements in user experience and engagement.
Meena's Architecture
Meena's architecture plays a critical role in understanding its capabilities as a conversational agent. The structure of an AI model is vital for defining how it processes and generates responses, shaping its ability to engage in meaningful dialogue. By examining the nuanced design of Meena, we can appreciate its potential to improve interactions in various applications, from personal assistants to customer service solutions. This section will delve into the elements that constitute its architecture, highlighting the advantages and considerations that come with such a design.
Technical Overview
Meena operates on a transformer architecture, which is pivotal in modern natural language processing models. Transformers are designed to handle sequential data effectively, allowing the model to retain context over longer conversations. This architecture employs a self-attention mechanism that allows Meena to weigh the importance of each word in a sentence relative to others, thus capturing intricate relationships and meanings.
The use of this architecture enables Meena to respond in more contextually relevant ways than many competitors. It discards the limitations of earlier models that relied heavily on fixed responses or simplistic generative techniques. In essence, the technical framework allows for a fluid exchange of information, ultimately supporting a more engaging dialogue.
Model Design and Features
Meena's design incorporates several key features that enhance its conversational abilities. Notably, it has 2.6 billion parameters, which provide it with a vast reservoir of knowledge from which to draw. This size is significant, as it translates into a deeper understanding of language nuances and topics.
Apart from its scale, Meena also includes an innovative classification model that stands out in determining the quality of responses. The model is trained to evaluate potential replies on multiple dimensions, such as engagement level and coherence. This not only assists Meena in selecting responses that are contextually appropriate but also enhances overall user satisfaction.
Another notable feature is the implementation of end-to-end training, which minimizes the need for extensive feature engineering. This seamless integration allows for a more streamlined training process where Meena learns to optimize its responses through real conversational interactions. As an outcome, the model becomes adept at handling a diverse array of topics while maintaining a conversational flow.
In summary, Meena's architecture is a sophisticated blend of innovative design and advanced technology that significantly improves the performance of conversational agents. The combination of its transformer-based approach, large parameter count, and effective classification mechanisms positions Meena as a powerful tool in the advancement of AI conversations.
Training Meena
Training is a crucial aspect of developing any conversational AI model. In the case of Meena, this process significantly influences its ability to generate human-like responses in varied contexts. The effectiveness of conversational agents heavily depends on the quality of training, which ties directly into dataset utilization and the techniques employed during the training phase.
Dataset Utilization
The dataset used for training Meena is a fundamental component. It provides the foundational knowledge from which the AI learns to understand and engage in conversations. Google designed Meena to leverage a diverse array of conversational data, allowing the model to grasp subtle nuances in language. This includes dialogues from social media, forums, and other public sources, thereby enriching its training pool.
Meena's creators recognized that the breadth of data is essential for achieving a high level of conversational fluency. They ensured that the dataset encompasses a variety of topics and styles. This not only helps Meena in understanding multiple contexts but also enhances its capability to respond appropriately in real-time interactions. The use of varied data sources minimizes biases and allows for a more rounded understanding of human language strands.
Moreover, the dataset's scale contributes to the model's ability to generalize learning. It can then effectively predict and produce relevant responses to a range of conversational prompts. Handling multiple threads of conversation simultaneously requires immense training, and a rich dataset serves as the ideal preparatory groundwork for this.
Training Techniques
The methods implemented in training Meena are equally notable. Google employed advanced techniques that prioritize end-to-end training, maximizing efficiency and performance. By utilizing a transformer-based architecture, Meena can interpret and generate language in a more context-aware manner.
Key techniques include:
- Supervised Learning: During this phase, the model learns from curated examples, specifically designed to improve its conversational skills. Supervised learning helps define the expected outputs for given inputs.
- Unsupervised Learning: This technique allows Meena to draw patterns from unlabeled data, which is crucial for developing its understanding of language semantics. It enables the model to adapt and improve continuously.
- Reinforcement Learning: Continuous optimization is achieved through feedback loops, where the AI is rewarded for generating accurate and relevant responses. This technique enhances Meena's ability over time.
A combination of these approaches results in a flexible and robust training mechanism. By balancing both supervised and unsupervised learning with reinforcement strategies, Meena can evolve, making it particularly adept at handling complex dialogues.
"The future of conversational AI lies in the training methods employed. Diverse data and robust techniques are key to development."
In summary, the training of Meena encapsulates a thorough approach, blending extensive datasets with advanced techniques. This holistic methodology ensures that Meena not only understands language but also learns to mimic conversational dynamics effectively.
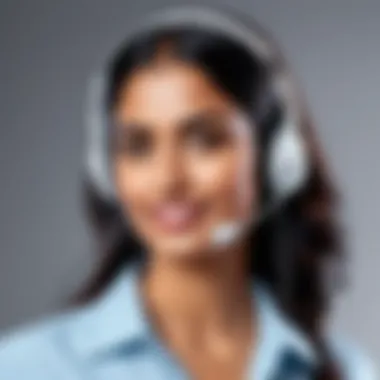
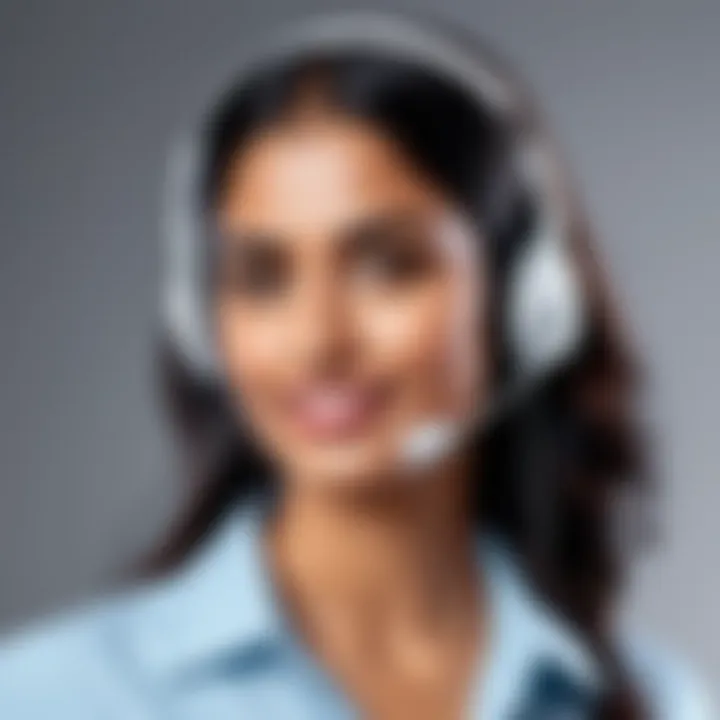
Performance Metrics of Meena
Assessing the performance of Meena is critical in understanding its capabilities and potential applications as a conversational agent. Performance metrics reveal how well the model interacts with users, providing insights into its strengths and weaknesses. Evaluating such metrics allows researchers and developers to make informed decisions regarding the integration and improvements of conversational AI.
Meena vs. Other Models
In the rapidly evolving field of conversational AI, comparing Meena with other existing models is essential. Some notable models include OpenAI's GPT-3 and Facebook's BlenderBot. Each of these models has distinct architectures and approaches to conversation. Meena distinguishes itself through its end-to-end training process, which enables it to generate contextually relevant responses across various topics.
A few key differentiators between Meena and other models are:
- Flexibility: Meena has an impressive ability to maintain context over extended interactions, which is crucial for meaningful conversations.
- Performance: Evaluations indicate Meena's conversational quality is on par with or exceeds that of its competitors.
- Open-domain capabilities: Unlike some models that might struggle with specific domains, Meena performs consistently across a wide range of open topics.
Meena's performance metrics not only reflect its conversational prowess but also highlight areas for future enhancements.
Quantitative Assessments
Quantitative assessments provide numerical data that can be analyzed for a clearer understanding of Meena's performance. Several metrics are commonly used to evaluate chatbot interactions, including perplexity, fluency scores, and user satisfaction ratings.
- Perplexity: This metric indicates how well a probability model predicts a sample. Lower perplexity scores suggest that Meena can better predict the next user input or conversational element.
- Fluency scores: These scores assess the naturalness of responses. High fluency scores imply that the conversational output sounds coherent and human-like.
- User satisfaction ratings: Collecting feedback from users through surveys and interactions helps gauge the effectiveness of Meena in a real-world context.
Evaluating these metrics allows developers to understand where Meena excels and where it may need improvements. These assessments play an important role when positioning Meena among contemporary conversational agents.
Use Cases for Meena
Meena is a transformational conversational AI model with practical applications that can significantly enhance various industries. Understanding the use cases for Meena allows companies to realize its potential. This section discusses how Meena can be integrated into customer service and personal assistant applications, showcasing its flexibility and utility.
Customer Service Applications
In today's fast-paced world, customer service is crucial for maintaining customer satisfaction and loyalty. Meena's ability to engage in natural and contextually relevant conversations makes it a powerful tool for customer support. Companies can deploy Meena in several ways:
- 24/7 Availability: Meena can handle inquiries any time of day. This constant availability ensures that customers receive assistance even outside regular business hours, improving overall satisfaction.
- Rapid Response Times: Meena processes inquiries quickly. The model's efficiency allows for faster resolution of customer issues, thereby reducing wait times.
- Scalability: In peak business periods, human agents can become overwhelmed. Meena can manage an increased volume of queries without compromising the quality of interactions. This scalability is vital for large organizations, especially during product launches or promotional seasons.
- Personalization: With its capacity to understand context, Meena can provide tailored responses based on user history and preferences. This level of personalization engages customers and creates a more satisfying experience.
As companies strive for operational efficiency and customer satisfaction, integrating Meena into customer service operations can lead to substantial improvements.
Integration in Personal Assistants
Meena's adaptability extends to personal assistant applications. Users are increasingly reliant on virtual assistants for everyday tasks. Meena enhances this experience through:
- Improved Conversational Context: Unlike many traditional assistants, Meena maintains context over multiple turns in conversation. This capability makes interactions feel more fluid and human-like.
- Task Management: By understanding user intent, Meena can assist users with scheduling, reminders, and other daily tasks effectively. This can streamline workflows and enhance productivity.
- Support for Complex Queries: Users often ask complex questions that require nuanced responses. Meena can handle such queries, providing users with detailed information rather than basic answers.
- Integration with IoT Devices: Meena can connect with smart devices, allowing users to control their environment through conversation. This integration makes daily tasks more convenient and intuitive.
The successful application of Meena in personal assistant technology can redefine how users interact with devices, leading to a more fulfilling experience.
"The future of AI lies not just in intelligence, but in the ability to converse naturally and meaningfully."
Challenges in Conversational AI
As we explore the realm of conversational AI, it is vital to address the myriad of challenges that impact the efficacy of these systems. Understanding these challenges not only illuminates the current limitations but also guides future developments in the field. The ability of systems like Meena to engage in meaningful conversations relies on overcoming these hurdles, which are both technical and ethical in nature.
Common Limitations
Despite substantial advancements in AI, conversational agents still face several innate limitations. One significant issue is contextual understanding. Many AI systems struggle to maintain context over extended conversations. When topics shift or when subtexts are involved, the ability to comprehend and respond appropriately diminishes.
Another prominent limitation is handling ambiguity. Humans often communicate with nuanced meanings, idioms, or even sarcasm. Conversational agents can misinterpret these subtleties, leading to responses that are either irrelevant or incorrect. For instance, a simple question could be redirected into an unexpected topic due to a lack of discernment in recognizing the intended meaning.

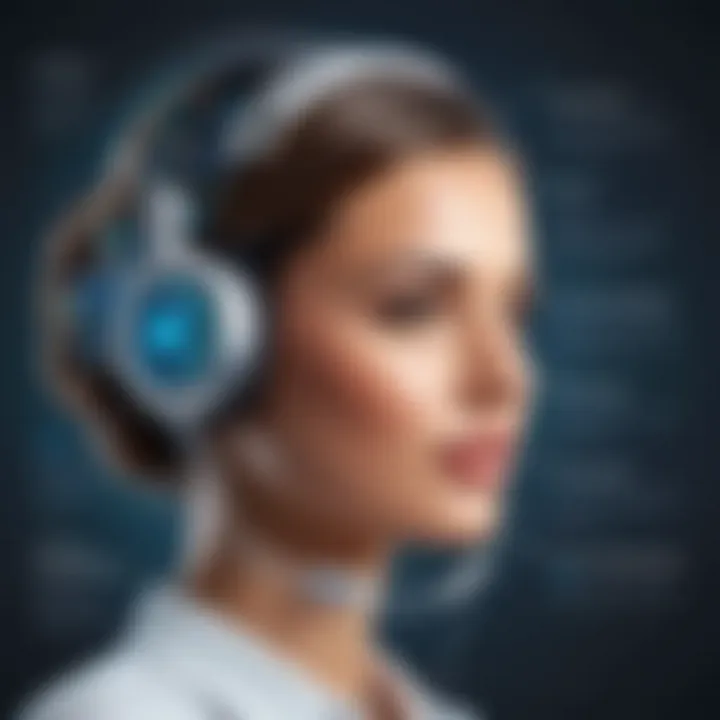
Furthermore, these models often rely on extensive data sets to improve their understanding. However, if the training data is not representative, biases can emerge in responses. This results in uneven performance across different demographic groups, further complicating the conversation quality.
- Technical Limitations: Context retention, ambiguous language handling.
- Data Bias: Performance variance based on training data distribution.
Ethical Considerations
Ethical considerations in conversational AI cannot be ignored as these systems become more integrated into daily life. One major concern revolves around privacy. Conversational agents often collect and analyze vast amounts of personal data for enhancements. This raises questions about consent and the safety of user information.
Moreover, the potential for misinformation is a significant ethical issue. If the AI provides incorrect or misleading information, it can lead to harmful consequences, especially in sensitive areas such as health or finance. Ensuring accuracy and reliability in responses is critical, yet complicated.
Another pressing concern is the psychological impact of human-like interactions. Users may form emotional attachments to their conversational agents, which can lead to dependency or distorted perceptions of relationships. This phenomenon may pose risks, particularly for vulnerable individuals.
- Privacy: Balancing data use and user confidentiality.
- Misinformation Risk: Potentially harmful effects of inaccurate responses.
- Psychological Dynamics: Emotional attachment and dependency on AI interactions.
"Conversational AI systems must tread carefully, balancing innovation with ethical integrity and user safety."
Ultimately, addressing these challenges in conversational AI is crucial not only for improving technology but also for ensuring that such advancements serve society responsibly. As we look to the future, understanding these complexities can drive more effective and ethical designs in conversational agents.
Future of Conversational Agents
The future of conversational agents represents a significant horizon for artificial intelligence technology. As systems continue to evolve, their potential to enhance user interaction becomes richer. This section will discuss potential advancements in conversational AI and their impact on society. An increasing number of industries are looking to integrate conversational agents. From customer support to personal digital assistants, the demand for natural dialogue interfaces is pressing.
Potential Advancements
Several advancements are likely to shape the future of conversational agents. These include improved natural language understanding, greater context awareness, and enhanced emotional intelligence.
- Natural Language Understanding (NLU): As NLU technology develops, conversational agents will better grasp nuances of human language. This means understanding idioms, context, and even sarcasm. This would allow for more meaningful and natural conversations.
- Context Awareness: Future systems could remember previous interactions and adapt responses based on this history. For instance, if a user frequently discusses technology topics, the agent could prioritize related information in future conversations.
- Emotional Intelligence: Advanced conversational agents will likely incorporate emotional recognition capabilities. This allows them to respond appropriately to user emotions. For example, if a user seems frustrated, the agent may alter its tone or offer reassurances.
Impact on Society
The implications of improving conversational agents can ripple across various facets of society. Users may benefit from more personalized interactions in their day-to-day activities.
- Enhanced Productivity: Conversational agents can manage schedule and task reminders, allowing individuals to focus on high-value activities. This improvement in efficiency could revolutionize the way people manage their time.
- Inclusion and Accessibility: Progress in conversational AI can make technology more accessible. Individuals with disabilities may find it easier to interact with devices. For instance, voice recognition allows hands-free operation, which is crucial for many users.
- Transformation of Job Roles: As conversational agents take over basic tasks, jobs may evolve. Employees may focus on more complex issues rather than routine inquiries. In some fields, this creates a need for new skills centered around managing AI systems.
Epilogue
The analysis of Meena provides substantial insights into its significance within the realm of conversational AI. This article emphasizes specific elements such as Meena's architecture and training methods, which highlight the critical advancements in natural language processing. Understanding these facets is vital for appreciating how Meena distinguishes itself from other conversational agents and its unique ability to engage in coherent open-domain conversations.
The benefits of Meena are manifold. It demonstrates how effective conversational agents can streamline interactions across various applications, such as customer support and personal assistance. By analyzing the performance metrics, we see how Meena's capabilities can enhance user experiences. Its success showcases an essential step toward future developments in AI technologies.
Moreover, the considerations surrounding the use of Meena remind us of the challenges posed by conversational agents. Ethical dilemmas, data privacy, and limitations are aspects that researchers must navigate. Thus, the conclusions drawn emphasize the need for continued vigilance and innovation in this field.
Summary of Insights
In summary, Meena symbolizes a remarkable stride in conversational AI, incorporating cutting-edge techniques to facilitate natural dialogues. Key insights from this exploration include:
- Meena's sophisticated architecture enables nuanced conversations.
- The extensive dataset utilized during training enhances its contextual understanding.
- Performance assessments illustrate Meena's competitive edge compared to other models.
- Significant applications in business sectors underscore the practical usefulness of conversational agents.
- Challenges such as ethical use and limitations remain focal points in future development.
These insights collectively aid in comprehending not only Meenaโs capabilities but also its relevance in shaping future interactions between humans and machines.
Future Directions for Research
Looking ahead, the research surrounding Meena and conversational AI opens many avenues. Future directions may include:
- Improving real-time responsiveness: Enhancements can lead to even more fluid conversations, making interactions seamless.
- Exploring multilingual capabilities: As our world becomes increasingly interconnected, focusing on language diversity can significantly impact the accessibility and reach of these agents.
- Addressing ethical concerns: Continued discourse on ethical frameworks in AI can ensure that advancements are responsible and beneficial to society at large.
Moreover, the intersection of Meena with emerging technologies, such as machine learning and augmented reality, could catalyze innovative applications that have not yet been imagined. Building upon this foundation, researchers must scrutinize and develop methods to create conversational agents that are not only efficient but also ethical and culturally aware.
Overall, Meena represents a pivotal moment in AI evolution, demanding a continuous and thoughtful exploration of its implications for the future.